AI’s potential meets data reality: Are you ready?
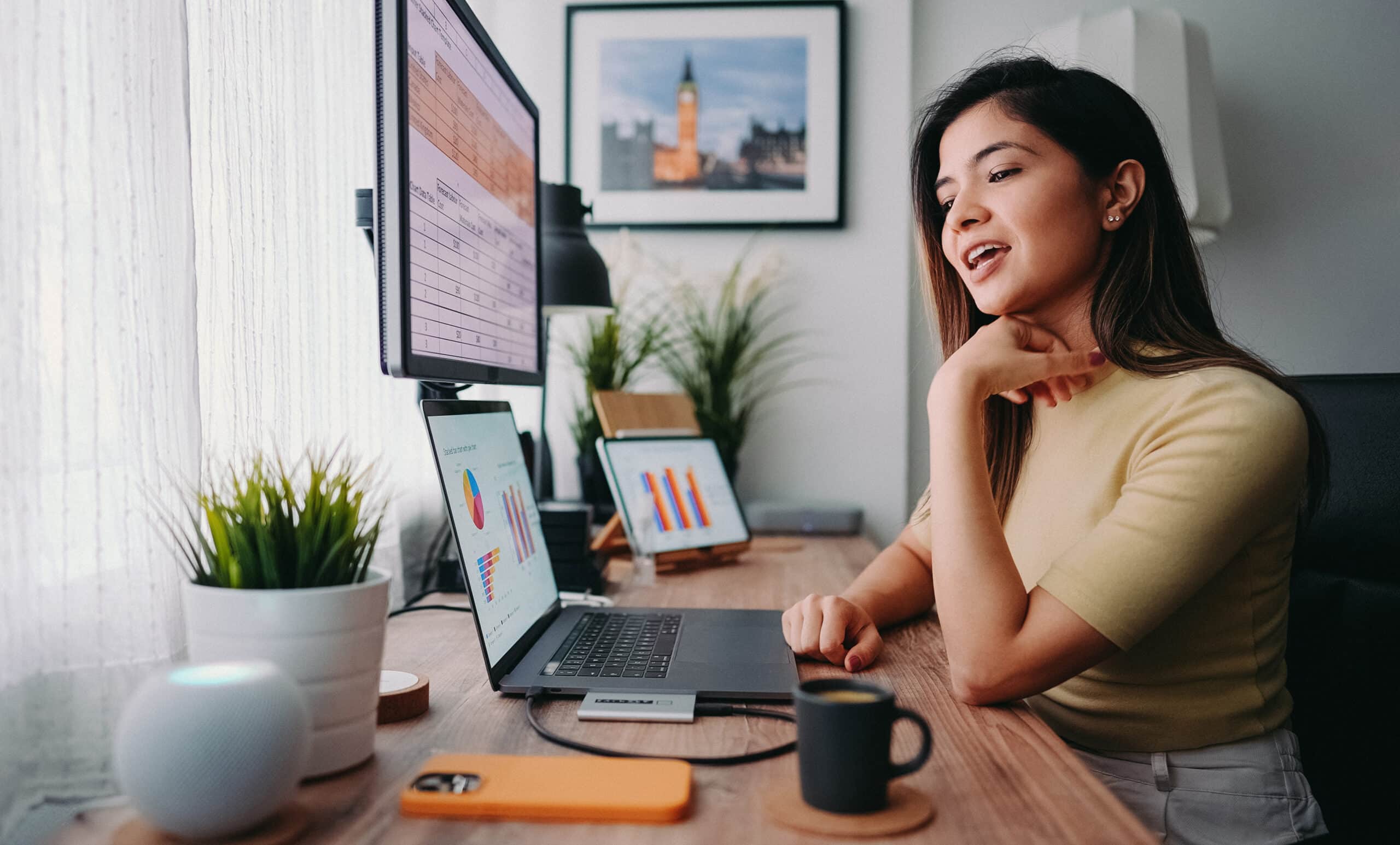
AI/ML is a “how” we solve hard technical problems. In other words, the tech has matured to the point where it is an essential tool in the toolkit for software, architects, developers, and testers. We also believe this mainstreaming and normalization of AI/ML technology is an industry-wide phenomenon. A straightforward approach to AI/ML is becoming essential for enterprises in every industry to remain competitive.
The potential for AI is awe-inspiring. McKinsey estimates, for example, that generative AI could add $2.6 to $4.4 trillion annually across 63 analyzed use cases in a recent study. By comparison, the United Kingdom’s entire GDP in 2021 was $3.1 trillion.
It’s no wonder then that a Workday survey found that 80% of senior leaders believe AI is required for their business to remain competitive. Similar to the cloud and digital transformation movements in recent years, AI tools are required for relevancy in the not-too-distant future. But leaders are finding that much like cloud and digital transformation – simply buying and implementing the latest tech and tools will not revolutionize your business. Poor data quality remains the biggest roadblock to realizing the game-changing potential of AI, and most organizations struggle to figure out why.
ROI on AI/ML relies on data quality
At the highest level, two problems must be resolved at the intersection of data quality and AI/ML. First, the data fed into model building is crucial for building trust inside and outside every organization. GenAI, LLMs, and other models used for business use need high-quality, trusted, compliant data that is updated in real time. Second, even the best models, once built, must act on incoming data and predict real-world outcomes. Untrustworthy, fragmented data that cannot be segmented or activated by humans effectively is unlikely to be actioned upon by ML models. That’s why organizations need a clear and comprehensive data strategy to lay the foundation for AI/ML. AI and ML investment ROI is greatly diminished without a coherent data strategy.
To be clear, we are not advocating that CDAOs solve every problem in data management before embarking on an AI/ML program–rather approach and set realistic expectations from AI/ML investments based on the maturity of your data program. Mature the data management approach in lockstep with the maturity of the AI/ML investments.
Is your data foundation ready for AI/ML?
Several key signals should be observed in determining your enterprise’s readiness for AI/ML adoption. Firstly, a data strategy should be well-defined, whether centralized, distributed, or hybrid. A clear understanding of critical dimensions is crucial; for instance, the term “customer” should have a universally accepted definition across the organization.
Next, data access rights should be transparent and governed effectively to ensure that only authorized personnel can access and modify the data. The state of the data is another vital signal; it should be cleansed and standardized to facilitate repeatable model building. This ensures that AI/ML models can be trained consistently and effectively.
Furthermore, reference data should be defined across the entire enterprise, ensuring a shared understanding and consistency in data interpretation. Cataloging data sources is essential, making data fully discoverable and easily accessible for AI/ML initiatives. Tracing both dimension and fact data back to their systems of origin is crucial for transparency and understanding the lineage of the data.
Uniform time-stamping of event and fact data is another significant indicator. This ensures that data is chronologically consistent, which is especially important for time-series analyses and other AI/ML applications that rely on temporal data. Lastly, the organization should have established norms around the ethical use of data. This ensures that AI/ML models are practical, ethically sound, and aligned with the organization’s values and societal expectations.
There’s no question that everyone will adopt AI programs, but the state of your data will determine their effectiveness. Understanding & communicating the potential outcomes of your AI program based on the quality of your data is a crucial starting point.
Asha Saxena, a successful entrepreneur and professor at Columbia University in business and medical schools, authored a compelling book called “The AI Factor.” In her book, she presents a data readiness framework (see the exhibit below), which provides a fantastic mental model for determining an organization’s preparedness for implementing AI models on top of an existing data foundation.
Building an AI strategy on a modern core data foundation
AI’s lofty promises to revolutionize business only come to fruition on a foundation of trustworthy data. Data – the fuel powering enterprise AI must be complete, accurate, and readily available. Modern cloud-native master data management (MDM) closes the trust gap by serving as the central nervous system for your business-critical core data.
The capabilities of modern MDM unlock AI’s full potential:
Single source of reliable data
Consolidating data from across siloed sources provides a “single source of the truth” for core data domains like customers, products, suppliers, and locations. This unified data acts as the training ground for accurate AI models. AI risks making dubious recommendations or failing to deliver for your customers without reliable core data.
Real-time data with low latency
Static data rapidly becomes stale. Modern MDM maintains master data by exchanging real-time updates across hundreds of systems. AI models can instantly incorporate new data via MDM’s APIs versus waiting for overnight batch jobs—this low latency access to trusted data powers intelligent “moment of need” interactions.
Rapid time to value
Lengthy development cycles delay AI benefits. With configurable MDM solutions on cloud platforms, organizations can launch centralized data management in weeks to months versus years. Pre-built connectors accelerate the ingesting and sharing of data across technology stacks. With quality data accessible from day one, data scientists can immediately focus on creating high-impact AI.
Flexibility and cost savings
Traditional, monolithic MDM systems with on-premises infrastructure create technical debt and inflexibility. Modern SaaS MDM enables starting small to prove value before scaling. Cloud deployment and usage-based pricing can contain costs. Open APIs and microservices architecture support changing needs. With affordable, adaptable MDM, AI innovation flourishes.
Modern MDM additionally incorporates AI/ML capabilities to enhance complex tasks, including entity resolution. AI/ML-trained models enable data consumers to quickly and accurately achieve value from customer, product, supplier, and other core business data domains. Unlike other solutions that require weeks of manual effort before ML recommendations are practical, an ML-powered entity resolution capability delivers instant value. Industry and domain-specific ML models are enabled with a click of a button.
Lay the data foundation to reap AI rewards
In today’s data-driven world, AI is mandatory to stay competitive. But artificial intelligence is only as strong as its data foundation. Modern MDM uniquely satisfies the needs of enterprise AI by curating complete, timely master data at scale. Treat your organization’s data as a strategic asset and invest in modern MDM to fuel AI with trusted data. Lay the data groundwork now to reap AI rewards for years to come.
Click here to watch a recent presentation on this topic.